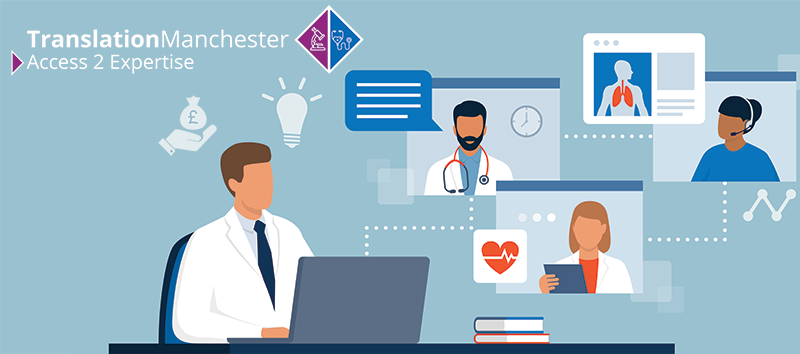
Access to Expertise (A2E) Awardees 2022
In 2022, Translation Mancheste run the first Translation Manchester Accelerator Awards (TMAA) call, bringing together funding from the Wellcome Translational Partnership Award (TPA) and the UKRI MRC Impact Accelerator Account (2022-25).
This funding call included two schemes; Confidence for Translation (C4T) and Access to Expertise (A2E). In particular, A2E provides up to £25,000 for researchers to access expertise external to their research group to overcome translational bottlenecks.
The call was extremely competitive with a great number of high quality proposals received for consideration. After thorough peer review the following proposals were funded:
The following A2E projects were funded through the Wellcome TPA:
Simon Harper
HypoCheat Clinical Trial Accelerator
Ellen Poliakoff
ACTION-PD: Delivering personalised video interventions by app for Parkinson’s and beyond
Claudia Lindner
Developing a technical and regulatory strategy to implement automated radiographic assessment into the Cerebral Palsy Integrated Pathway programme.
Caroline Jay
ECG-X: Explainable automated ECG interpretation for Long QT Syndrome – route to market
Martin Baron
Synthetic lethal targeting of Notch-driven breast cancer
These studies join our strong portfolio of translational research projects, spanning across the full translational pathway, which we are currently supporting in order to facilitate their journey towards patient benefit.
A lay summary for each project can be found below:
Martin Baron
Synthetic lethal targeting of Notch-driven breast cancer
Cancer is a disease caused by misregulation of signals that regulate cell fate, proliferation and survival. Often these are the same signals that control embryo development of organisms, and cellular turnover, replacement and repair of tissues in the adult. Modern drug discovery programmes for novel cancer therapies are attempting to target these misregulated signals. Ultimately the goal is personalised treatment that first identifies the key aberrant signal driving an individuals cancer and then utilises a medicine that switches off that signal. A problem with this approach is that many cells across different tissues use the same signals to control normal cell processes and so such approaches are likely produce side effects that reduces the ability to target the disease specifically. One example of this is the Notch pathway. Notch is the name of a cell surface receptor which, when activated, controls cell fate decisions, proliferation and cell survival. It is misactivated in many cancers including breast cancer. Attempts to target Notch have revealed difficult side effects, which hamper the ability to safely target the cancer. In this project we are developing a different approach. Our aim is to identify processes in the cell that become critical to cell survival only when Notch is over activated. This will generate drug targets, which are safe to use for normal tissues, i.e. the treatment would have little effect in normal tissues, but would kill cancer cell which have over activated Notch. To identify these we will screen the entire expressed human genome in cells in which Notch signalling is low and in the same when we switch on activated Notch. We have obtained proof of principle for this approach in Drosophila cells and now we will translate the methodology to a human breast cell line which becomes cancerous when Notch is activated in it. We expect the programme to identify genes whose function is critical to the cell only in the cancerous state to be carried forward to the next step in the drug discovery pipeline.
Ellen Poliakoff
ACTION-PD: Delivering personalised video interventions by app for Parkinson’s and beyond
Parkinson’s disease (PD) affects approximately 1 in 500 people, causing significant difficulties with mobility and activities of daily living. The ability to control fine hand movements (dexterity) needed for everyday actions such as dressing, writing, and preparing food, is affected in Parkinson’s. This reduces people’s independence and quality of life and has been identified as a top research priority by people living with Parkinson’s. However, there is little research into treatment options for dexterity. Our vision is to create an app for people with Parkinson’s to train at home to improve their hand movements for everyday actions. The training involves watching videos, imagining, and copying movements. To make the app and training as useful and usable as possible, we have worked with people with Parkinson’s and physiotherapists on its design.
We have tried out a prototype version of the app in a small study, which showed that people with Parkinson’s found the app and training acceptable and usable. Before testing the app and training in a trial to see if it can improve everyday actions, we need to develop a fully functioning app. This will also allow us to personalise and adapt the training for people, so it is appropriate and challenging enough for them. In this stage of the project, we will develop the app to be able to deliver personalised training programmes incorporating videos and instructions. The app will also be designed in a way that it can be used to deliver training for people with other health conditions in the future.
Simon Harper
HypoCheat Clinical Trial Accelerator
Congenital Hyperinsulinism (CHI) is a condition with causes exceptionally low blood sugar in children, which in turn produces brain damage in up to 48% of those effected (costing the NHS in excess of £3.4million/year).
Current medical management of CHI is insufficient to prevent hypoglycaemia and thus methods of prevention utilising glucose monitoring and hypoglycaemia prediction are required. Blood Glucose sensing has emerged as a method of hypoglycaemia prediction This approach is undermined by poor sensing accuracy in patients with CHI and has failed to demonstrate any real-world reduction.
We have developed an alternative approach that automatically aggregates sensor data into a succinct and easy to understand ‘heatmap’ of weekly hypoglycaemia risk. Our work outperforms proprietary software in the identification of hypoglycaemia patterns. Furthermore, the patterns identified are associated with repetitive behaviours that are targeted by our persuasive technology to ensure that patterns are translated into proactive hypoglycaemia prevention.
This has produced a software prototype which, when tested in pilot studies, reduces low blood sugar (hypoglycaemia) ‘hotspots’ by 67% and total hypoglycaemia by 25%. Larger studies are now needed to validate our initial success.
However, our prototype requires a high amount of researcher support, which is not sustainable for these larger studies. In this case we need to re-engineer the prototype to remove this reliance on researcher support and make the application scale to a much larger cohort. Simply, we need to optimize the system and this application is to do just that.
Caroline Jay
ECG-X: Explainable automated ECG interpretation for Long QT Syndrome – route to market
In a busy hospital, a junior A&E doctor is learning to interpret ECGs. Where once she would have been counting tiny squares and trying to manually determine the beginning and end of waves using trigonometry – for example drawing tangents – on a paper printout, instead she is exploring the data on an iPad, changing the axes and orientation of the ECG, applying colour to the area under the curve and zooming in to understand details of the signal. The visualisation tools she can use to understand the data also support her in another way: providing an automated estimation of the likelihood of the conditions she is investigating, explaining in natural language how it has come to its judgement. Because the automated interpretation uses ‘cognitive fit’ – where the human and the machine share the same representation of the data – it is intuitively understandable, and easy to check and explore. In this case, the fit is provided by the visual presentation of the ECG data. The techniques that support human interpretation, which combine pre-attentive processing to highlight anomalies in the signal with clinical knowledge to ensure reliability and accuracy, are also used as the basis for machine interpretation.
The Electrocardiogram (ECG) is a graphical representation of the heart’s electrical activity that is widely used in clinical practice for detecting cardiac pathologies. ECG interpretation is highly complex, challenging both humans and machines. Our research is making this much more straightforward, meaning that in the future both clinicians for both clinicians and lay people will be able to understand ECG data.
This project is looking at one particular condition – long QT syndrome – which arises when the heart takes slightly too long to recharge after it has pumped blood round the body. It is caused by many common medications and can be fatal. It is the leading cause of sudden cardiac death in people under the age of 40, and is often the reason that athletes or footballers suddenly collapse while on the field. Our algorithm can tell whether someone is at risk of long QT syndrome from monitoring their ECG, and the risk can be visualised using a rainbow of colour on each heartbeat. The project will be examining how we should take this forward, and in particular whether we should focus on self-monitoring, using wearable devices, or whether we should be integrating the technology into hospital settings.
Claudia Lindner
Developing a technical and regulatory strategy to implement automated radiographic assessment into the Cerebral Palsy Integrated Pathway programme
Cerebral Palsy (CP) is the most common motor disability in childhood. Affected children are at high risk of hip dislocation – where the ball of the joint moves out of the socket in the pelvis. This leads to severe pain and difficulties with personal care. Hip dislocation can be prevented if signs are spotted early (when the ball begins to move out of the socket) and are appropriately treated.
A national programme called Cerebral Palsy Integrated Pathways (CPIPs) is being rolled out to monitor the hips of children with CP. As part of CPIPs, hip X-rays are taken as frequently as every six month and clinical experts use the X-ray images to manually take measurements that allow them to monitor the position of the ball in the socket. However, taking these measurements manually (i) takes a lot of time – in particular as part of a national programme such as CPIPs; (ii) is prone to errors; and (iii) adds delays.
We have developed a computer tool to automatically calculate the measurements used to identify hip dislocation. We have shown that our tool takes measurements that are similar to those taken manually by clinical experts. Our goal is to get the tool into the hospitals such that every child with CP can benefit from their hips being monitored. The results of the tool would always be checked by a clinician.
In this project, we will work with experts from industry to prepare a plan on how to extend the tool so that it can be easily used by doctors in hospitals, and to understand which approvals are required before it can be integrated into the healthcare system.
0 Comments